Research Group Prof. Geiß – Georisk Research using Remote Sensing Methods (in cooperation with the German Aerospace Center)
The research group for Remote Sensing Techniques for Natural Hazard Risk Assessment focuses on the development of novel Machine Learning/Artificial Intelligence methods, earth observation techniques, and natural hazard risk and impact assessment.
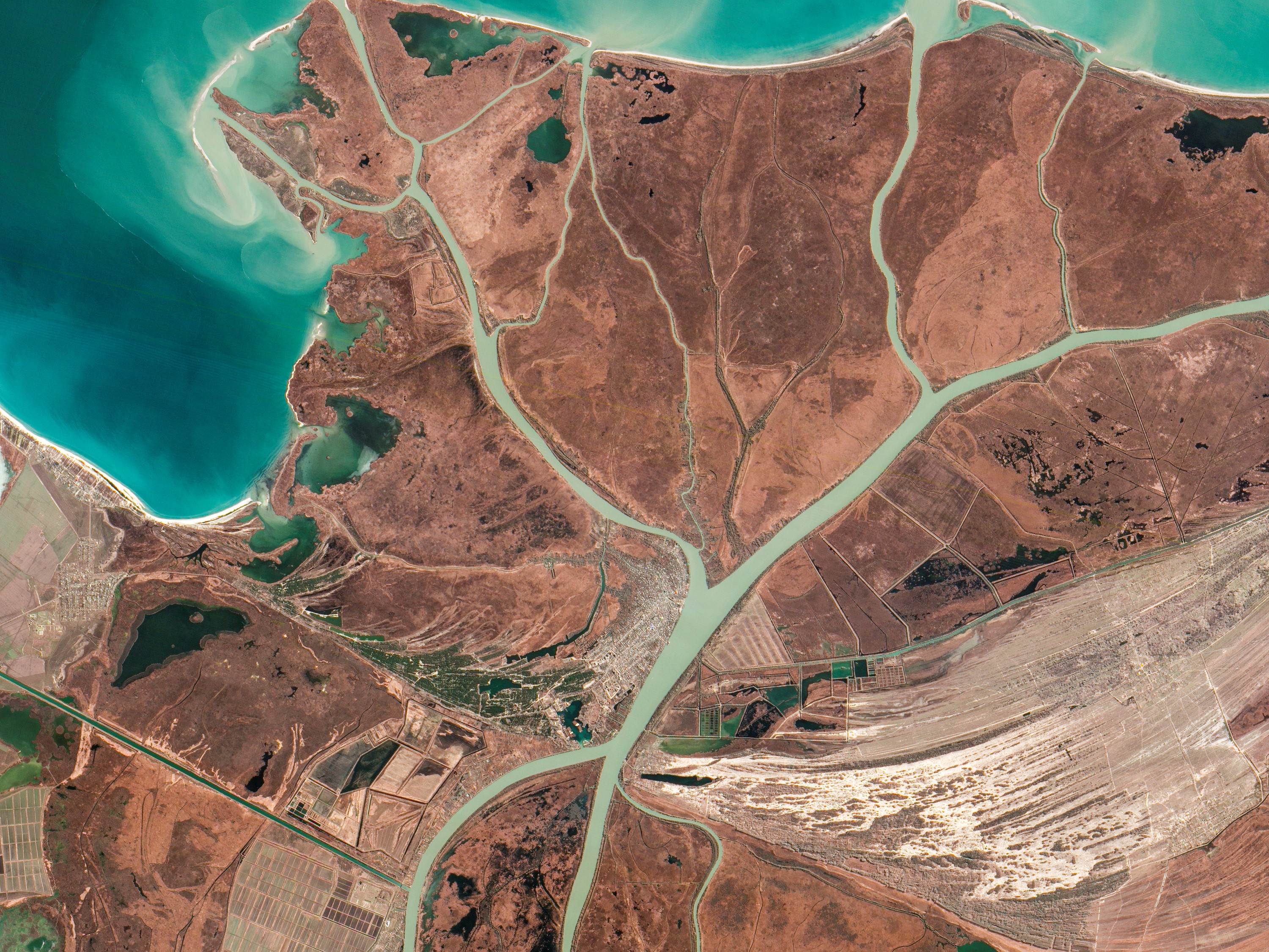
Machine Learning and Georisk
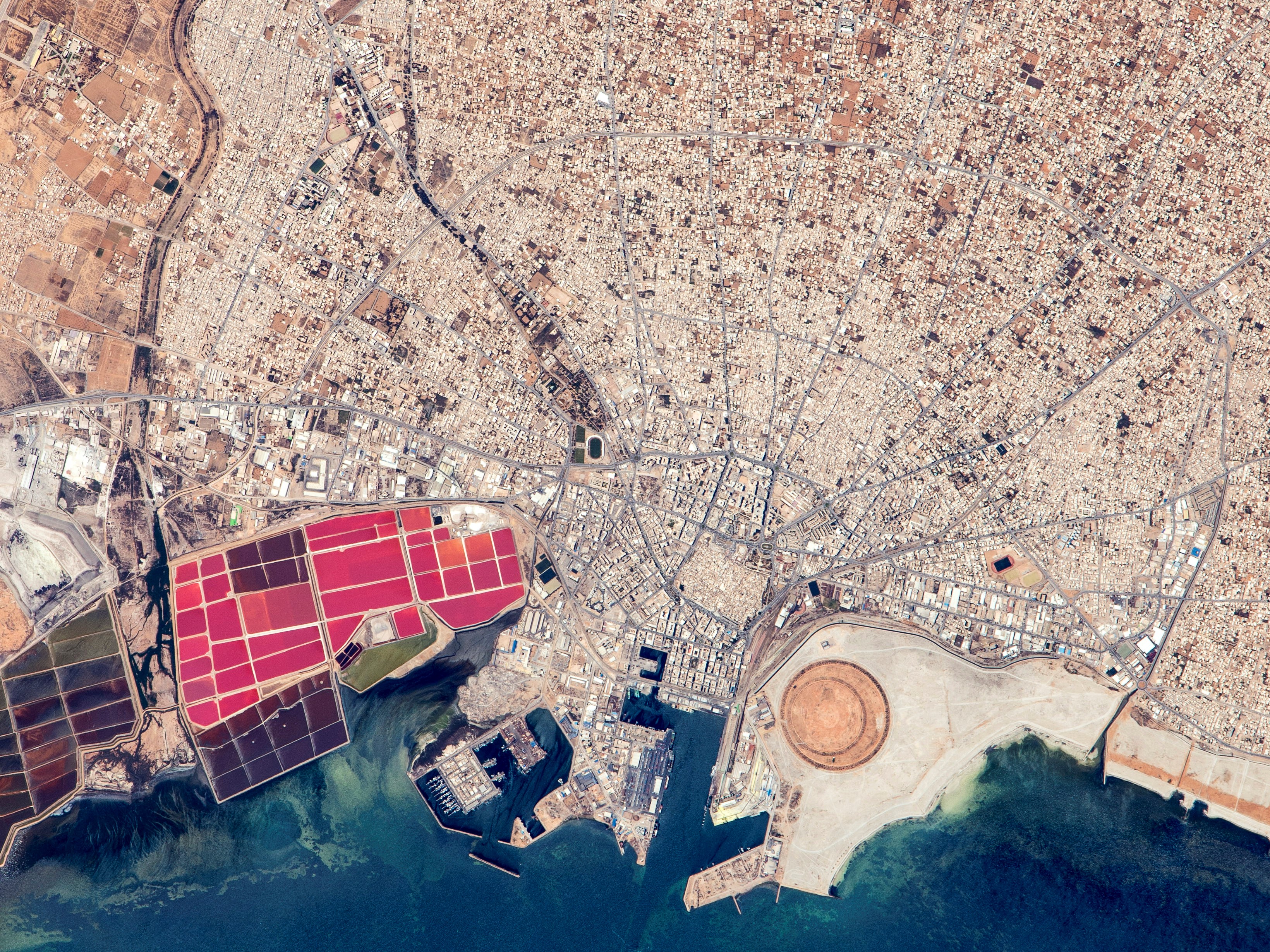
Research
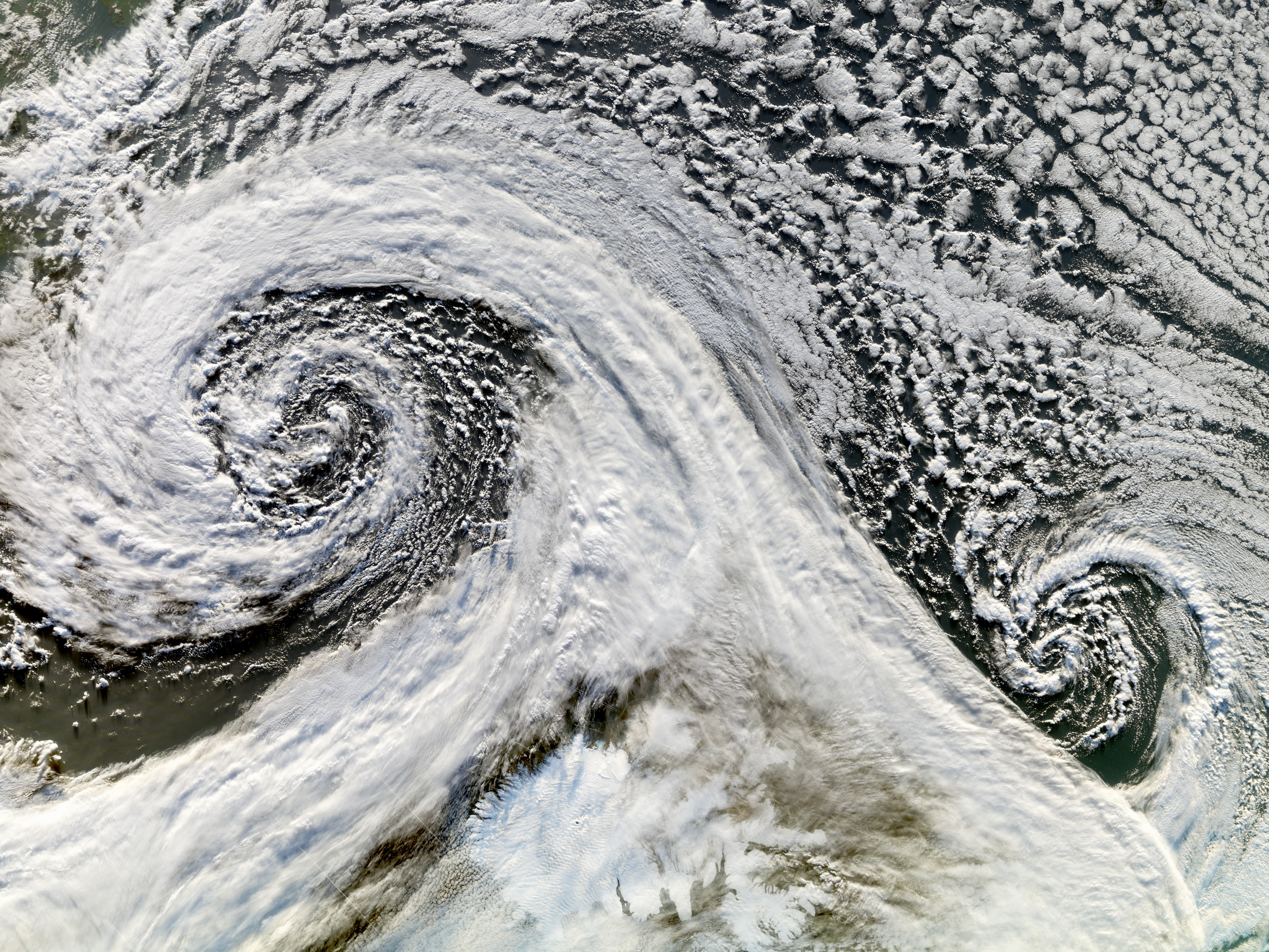
Prof. Christian Geiß
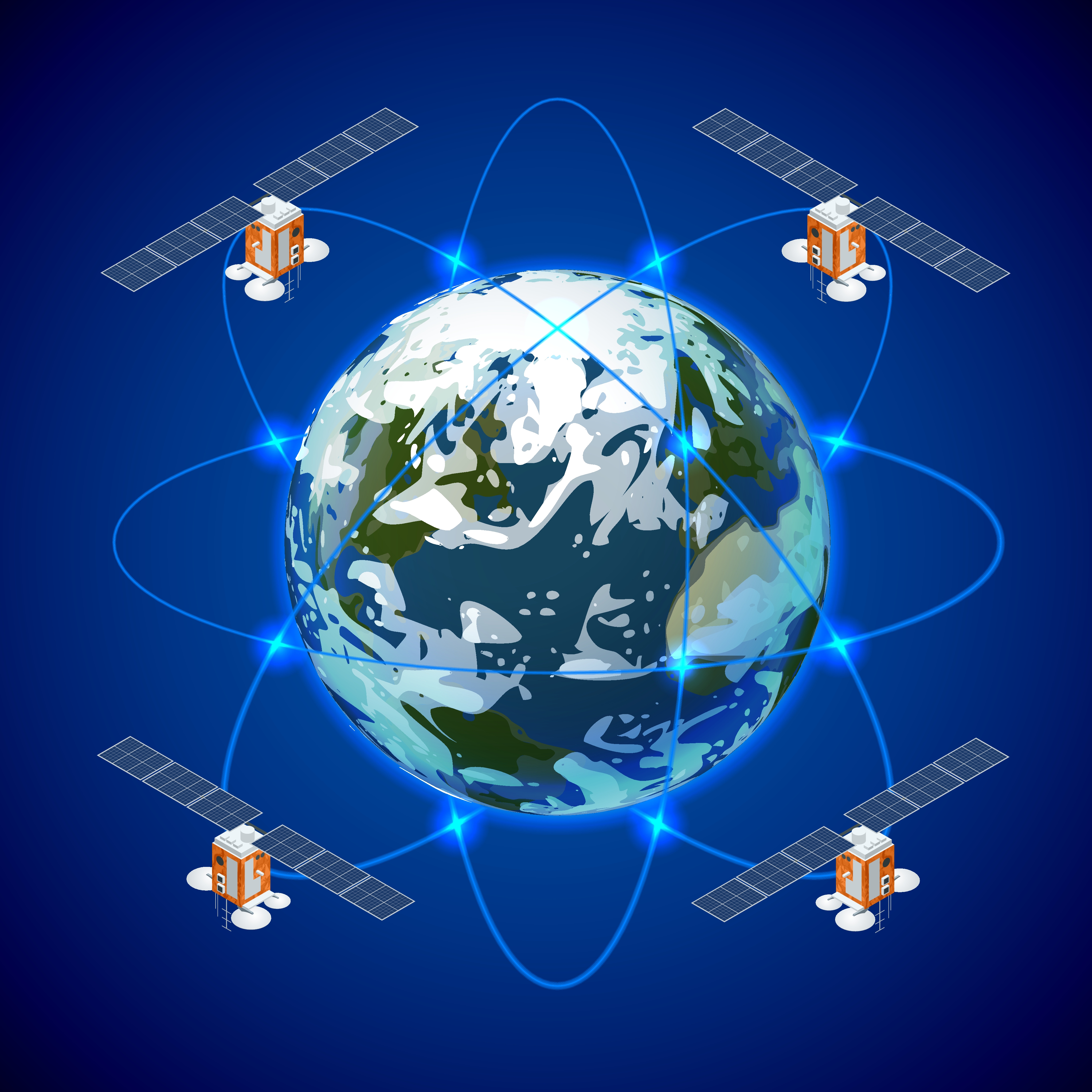
Global change encompasses human-driven changes to the Earth’s system. These changes include increasing urbanization and increasing exposure and vulnerability to natural hazards.
Concerning the quantitative representation of natural hazard-related risks, a multitude of research needs arise. Existing risk models often solely allow a very coarse estimation of risks, as they are based on strongly aggregated or outdated input data. This situation is increasingly aggravated as urbanization processes dramatically reshape built environments within a very short time frame. Accordingly, it is currently almost impossible to accurately quantify and anticipate the risks and potential consequences of natural hazards such as earthquakes, floods, and storms from a global perspective. To significantly improve the understanding in this regard, one of the main goals of the working group is to provide new data on the risk-related properties of the built environment (exposure information) for risk models on a global scale, ensuring high spatial, thematic, and temporal resolution.For this purpose, it is necessary to develop new algorithms and techniques to synergistically analyze constantly growing geodata sets. These data sets include remote sensing missions providing global land surface observation data (e.g., TanDEM-X, Sentinel-1 & 2), ground-based imagery data (e.g., Google Street View), geolocated data from social media (e.g., Twitter), and geographic information collected by volunteers (VGI), among others. A particular focus of the working group comprises the development of new machine learning methods/artificial intelligence techniques to extract thematic information from the aforementioned heterogeneous data sources with high accuracy. Finally, the data and techniques are applied to assess the exposure and vulnerability of built environments around the globe for anticipation of future damage, to inform local populations and authorities about their current level of risk, as well as to implement appropriate mitigation measures.
The main research areas comprise:
- multimodal remote sensing of the built environment
- the development of machine learning methods for the interpretation of earth observation data
- exposure and vulnerability assessment in the context of natural hazards
- techniques for automated damage assessment following natural disasters
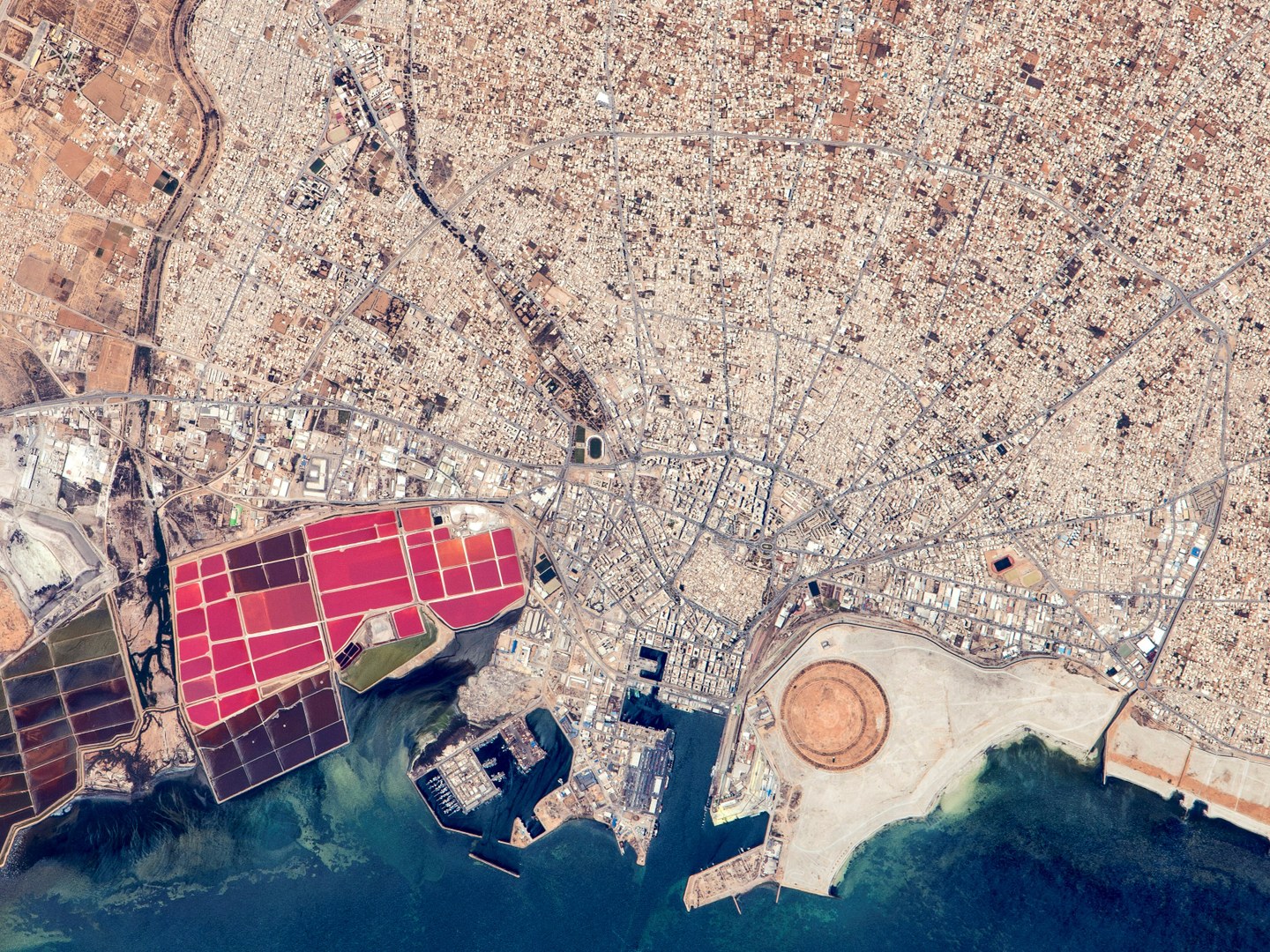
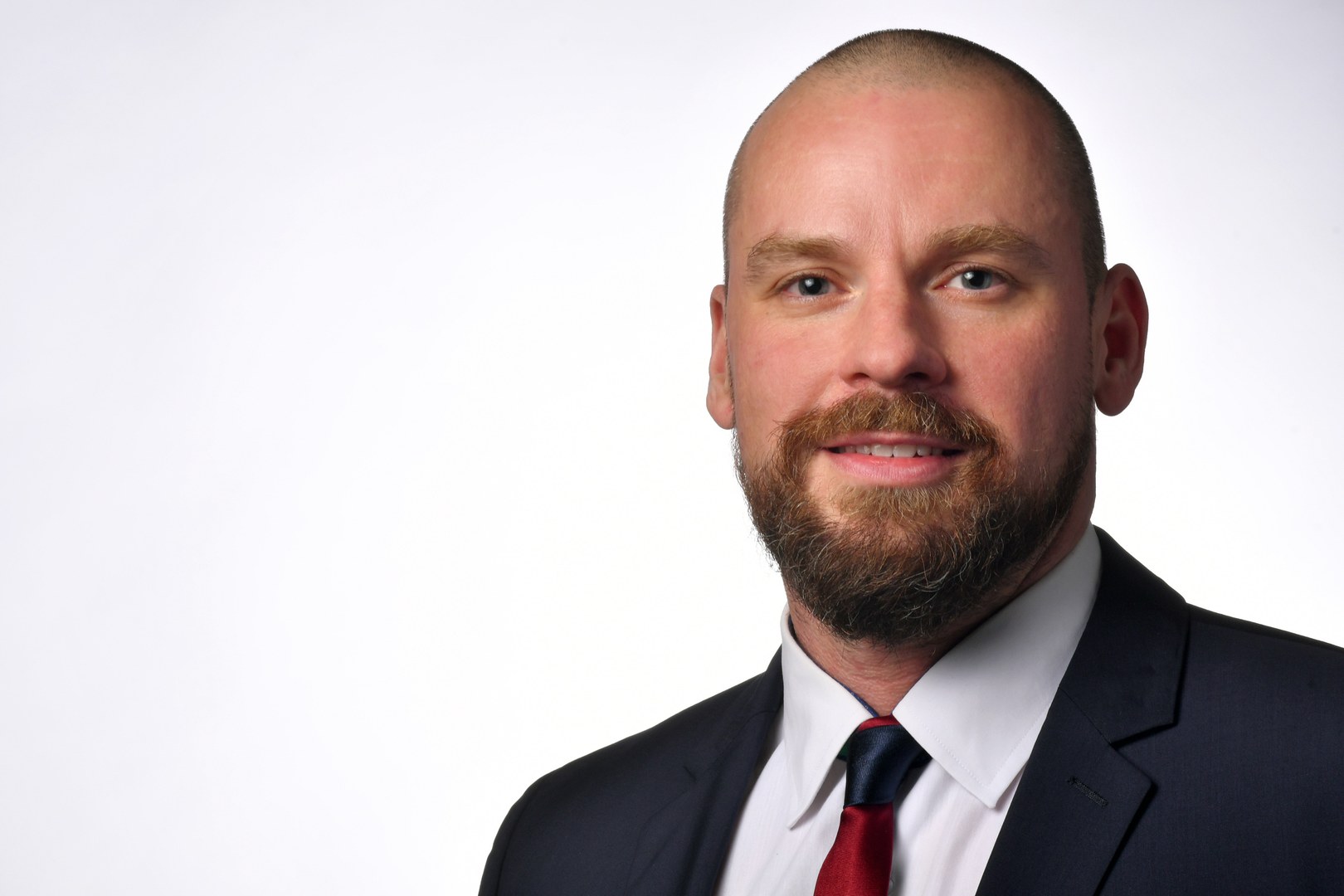
Prof. Christian Geiß
To learn more about me, please refer to my CV, available on the website of the German Aerospace Center (DLR).
Teaching activities comprise:
- Machine Learning/Artificial Intelligence methods
- Remote Sensing 4 Natural Hazard Risk Assessment
- Scientific writing
Selected Publications
Contact
Prof. Dr. Christian Geiß
2.002 (342)